Artificial intelligence (AI) and machine learning (ML) are hot topics in technology. New use cases and applications are discussed daily—from search results recommendations to smart cars. But what are cybersecurity organizations doing with this tech? What does it take to render additional security out of AI? And how do AI and ML change the way we fight cybercrime?
Both AI and ML are already being adopted and implemented in many cybersecurity platforms. But before these two forms of tech achieve mainstream traction, it’s important to discuss their impacts.
The difference between AI and ML
The problem with hot tech like artificial intelligence and machine learning is that people and companies end up having different perceptions of what they really are. As to not muddy the water, let’s start by explaining the relationship between the two. Artificial intelligence and machine learning are not interchangeable. Consider machine learning, instead, as a sort of offspring of artificial intelligence.
Artificial intelligence is achieved when machines carry out tasks that are not pre-programmed and in a way that we consider “smart.” Take, for example, a computer that can play chess. There is a big difference between a chess computer that has countless situations pre-programmed and performs the given solution, or a chess computer that analyzes the position of the pieces and calculates the outcome for every possible move many moves ahead. The first is executing commands. The second is using artificial intelligence.
Machine learning is an algorithm that, when fed enough information, is capable of recognizing patterns in new data and learning to classify that new data based on the information it already has. Essentially, these algorithms teach the machine how to learn. An apparent danger in this method is that if the machine is allowed to accept its own assumptions to be true, it may stray from the path the developers envisioned.
To sum it up, AI focuses on building smart machines while ML is about creating the algorithms that allow machines to learn from experience.
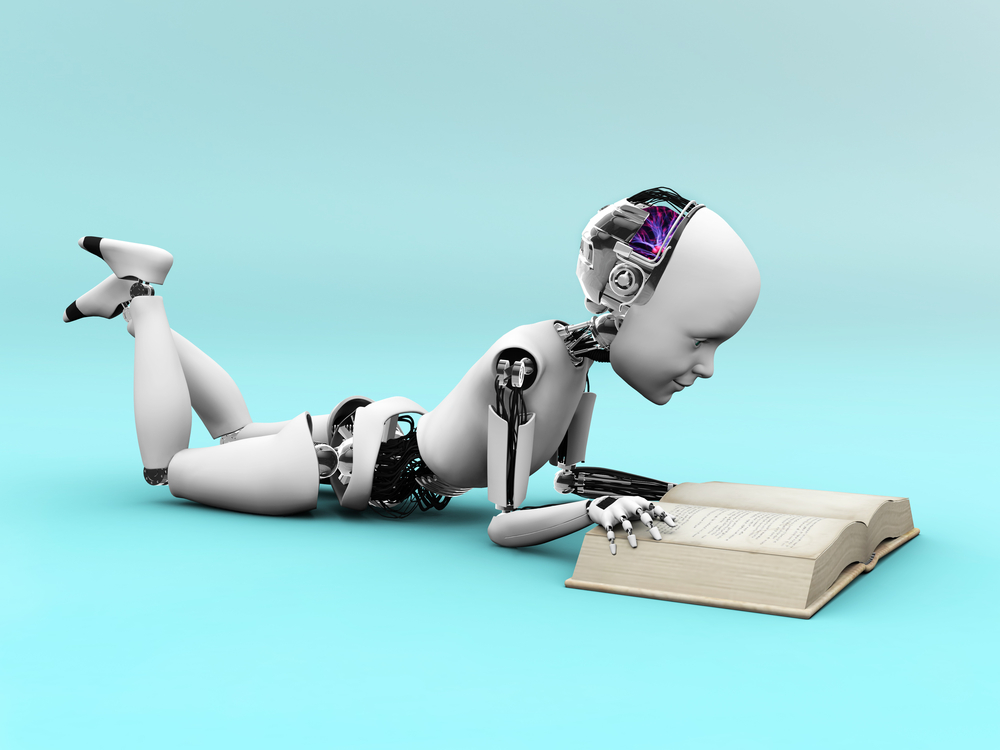
Examples of AI and ML
We have all seen examples of AI/ML in our daily lives. Some of them we may recognize as such, but others have become so common, that we don’t even notice them anymore. Autofill, for example—a tool we’ve become accustomed to in search engines, SMS messages, and chat applications—would never exist without machine learning. (Because the machine learns what your next word is likely to be and suggests it.)In some areas, we have seen lots of progress in artificial intelligence and machine learning: self-driving cars, voice recognition, image analysis, and medical devices. And, as referenced in Minority Report, AI has many applications in the field of targeted marketing/ personalized advertisements.
Current use in cybersecurity
If it weren’t for developments in AI and ML, it would be much harder for cybersecurity companies to detect all the new malware that comes to the surface every day. Therefore, it makes sense to use the options offered by these fast-growing fields to our advantage. At Malwarebytes, we already use a machine learning component that detects malware that’s never been seen before in the wild (zero-days). And other components of our software perform behavior-based, heuristic detections—meaning they may not recognize a particular code as malicious, but they have determined that a file or website is acting in a way that it shouldn’t. This tech is also based on AI/ML.
Other so-called “next-gen” security solutions promise to protect their customers against zero-days and ransomware in a similar way, so there does seem to be a trend in this with some of the newer cybersecurity companies. But not all of them call their methods “artificial intelligence” or “machine learning,” which makes it hard to determine how mainstream they really are in cybersecurity.
It seems that while most companies might be well aware of the need for new security strategies, not many have implemented AI/ML to fight back against the rising tide of ever-more sophisticated malware.
Perfect fit
Let’s face it: Machines are much better and more cost-efficient than humans when it comes to handling huge amounts of data and performing routine tasks. This is exactly what the cybersecurity industry needs at the moment, especially with large amounts of new threats appearing every day.
Most of these new threats can easily be classified into existing families or familiar types of threats. In most cases, spending time looking over each new threat in detail would be a waste of time for a researcher or reverse engineer. Human classification, especially in bulk, will be error-prone due to boredom and distractions. Machines, however, do not mind going through the same routine over and over, and they do it much faster and more efficiently than people do.
But that doesn’t mean they always get it right. Even with an AI, it will be necessary to keep an eye on the work to check whether the algorithms are still working within the desired parameters. AI and ML without human interference might drift from the set path. But with an AI as a partner, researchers needn’t be buried in menial work.
Classification and…?
How else can we use AI and ML in cybersecurity? In fact, anything can be used as a basis for a machine learning algorithm as long as you have enough data on it to detect a pattern that leads to accurate conclusions.
Take, for example, attribution. Right now, it’s quite difficult for security researchers to determine who was behind an attack. They must take the forensic artifacts of a cyberattack and match them to known threats against targets with similar profiles. Or in other words, try to figure out the attacker based on the methods used and who the target was (or might have been).
Now, it’s anyone’s guess who was behind an attack, and fingers are often pointed in convenient directions (It was the Russians!) instead of accurate ones. But with the help of artificial intelligence and machine learning, we can pinpoint the origin of the attack with more accuracy.
Machine learning can also be used for security projects outside of infosec. For example, the UK government has selected eight machine learning projects to boost airport security. The selected projects will make use of ML techniques to detect threats on passengers and in bags, like an imaging device that can scan shoes for explosive materials. This effort is meant to shorten the time spent by passengers in queues during their screening process.
Applying machine learning to security efforts, even those outside of cybersecurity, offers both those charged with keeping the world secure and those looking for protection a solution that sacrifices neither accuracy nor efficiency.
Adversarial machine learning
One of the reasons why we will want human checks on the development of the ML algorithms and their results is the unavoidable coming of adversarial machine learning. In a nutshell, adversarial machine learning means the “bad guys” will come up with ways to lead our AL or ML astray. In cybersecurity, this could result in confusing the detection routines to a point where they would allow malware through. This is one of the reasons to use AI and ML alongside more traditional detection methods. When considering implementing artificial intelligence or machine learning, creating a system of checks and balances can help put to rest fears that the technology will be abused for wrongdoing.
Summary
Artificial intelligence and machine learning have already gained a foothold in cybersecurity, but we can promise you that this development will go a long way further as the two fields are a perfect fit. The amounts of new data coming in every day are too much for cost-effective human processing, and machines are less error-prone if trained properly. There will be some kinks to work out, as AI and ML are still very much in development phases. The expectation is that the implementation of AI and ML will make the human work less in quantity but more challenging.